Development of a Jos cardiovascular disease risk app to improve screening for atherosclerotic cardiovascular diseases
Introduction
It has been said that assessing risk of cardiovascular disease (CVD) is a necessary first step in preventive cardiology; and that baseline atherosclerotic cardiovascular disease (ASCVD) risk assessments are better in predicting those who will benefit from treatment (1). Previously, population studies have documented these CVD risk factors imputing them in multi-variate risk scores to predict ASCVD (2). These various scores were derived from risk equations acquired from populations with limited ethnic diversity. That some of them are sub-optimal (3) needs to be acknowledged, and research efforts designed to improve risk assessment in asymptomatic at-risk population ramped up (1). This need prompted the Koreans to come up with a prediction model appropriate to their ethnic group (4). Recognising the limitations inherent with these algorithms, some additional indices have been recommended to refine the risk estimates. The currently identified ones in the words of Kullo et al. (1) did not significantly improve prediction of 10-year risk of ASCVD. Recently, we found that abdominal height (AH) as measured by a locally conceptualized appliance, the Abdominometer predicted cardiometabolic diseases better than previously established anthropometric indices (5). This was recently confirmed further, in a large population of free-living rural dwellers in North-Central Nigeria (6).
It has been posited that populations on which current ASCVD risk scores are based have few African- Americans, implying need for re-calibration when used in non-US populations. Consequently, it was recommended that at intervals, new algorithms should be developed to take care of this inadequacy (7). Using existing algorithms, the MESA study found that up to 60% of cardiovascular events occurred in individuals not classified as high risk by either traditional risk factors or even the newer coronary artery calcium score (8). The implication is that existing algorithms are rather low in sensitivity.
We have therefore decided to introduce AH and body surface index (BSI) into the traditional CVD risk factors to develop an ASCVD prediction tool for CVD local to us in sub-Saharan Africa.
The factors considered were carefully chosen to avoid phlebotomy and biochemical assays that may come with a cost in our environment. We then compared this tool with sub-clinical atherosclerosis confirmed on carotid intima media thickness (CIMT) (a standard measure of atherosclerosis) and the widely used Framingham Risk Score (FRS).
Methods
This study secondarily analysed data generated as part of a larger study we undertook on sub-clinical atherosclerosis in apparently normal individuals. Apparently healthy (not known to have any cardio metabolic disease or on related treatment) adult Nigerians in Jos were invited to join the study from among senior and junior staff of Jos University Teaching Hospital as well as their relations; and students on various postings in the hospital. The minimum sample size was calculated to be 196, but was increased to 221 to improve on power. Adults above 18 years were included but pregnancy and puerperium served as exclusion criteria. The study was conducted in accordance with the Declaration of Helsinki (as revised in 2013) and was approved by the Ethics and Research Committee of Jos University Teaching Hospital, Jos Nigeria (Registration Number: NHREC/13/03/2010) in a memo JUTH/DCS/ADM/127/XIXI/6257 of 29th July 2015. Informed consent was taken from all the subjects.
Demographic, behavioural and other risk factor information needed for the study were obtained by administering a questionnaire by one of the authors; with emphasis on gender, family history of CVD alcohol use, physical inactivity and cigarette smoking. They then underwent a physical examination. Body weight (w) was measured with a Hana Bathroom weighing scale to the nearest 0.5 kg with subjects wearing light clothing; and no shoes, with pockets emptied of all items. The scale was corrected for zero error before each measurement. The height (h) was measured in centimeters to the nearest 0.1 cm against a stadiometer without foot wear or head gear. BSI was derived from the formula body weight (kg) divided by body surface area (m2) as enunciated by Ferreira and Duarte (9), where body surface area equals [weight (kg) × height (cm)/3,600]1/2 AH was measured using the locally constructed appliance called the Abdominometer in the fashion enunciated by the inventor and published previously (10). Systolic blood pressure (SBP) and diastolic blood pressure (DBP) were measured with the digital blood pressure monitor (OMRON M2 Basic). The subjects sat quietly for about 15 min after which the adult cuff was applied on the left upper arm resting on a table at the level of the heart. The appliance was then activated. The measurements were repeated twice at 5 min intervals. The average of the last 2 measurements was taken as measures of SBP and DBP. For vascular interrogation of the carotid artery, we used the Logic 5 Expert Duplex Ultrasound Scanner (GE Medical Systems, USA) fixed with a 7.5 MHz linear transducer. The details have been published elsewhere (10), but essentially subjects were positioned appropriately to access the right common carotid artery. The ultrasound transmission gel was applied to the neck and the transducer placed on it. The CIMT was measured as the distance between the intima and media layers of the carotid artery wall. The average of 2 measurements was used for analysis. A single qualified and experienced consultant radiologist carried out all the diagnostic ultrasound measurements.
The algorithm for determining sub-clinical atherosclerosis risk utilized the IDENTIFY RISK paradigm with 7 steps. The algorithm utilized 10 risk factors or parameters namely: age, AH, BSI, SBP, DBP, gender, family history of cardiovascular disease (FH CVD), alcohol use, physical inactivity (PI) and smoking. Thresholds corresponding to sub-clinical atherosclerosis using CIMT were obtained from ROC curve analysis utilizing data from the main study as determined earlier and assigned points as shown in Table 1. The IDENTIFY RISK () algorithm identifies and classifies the sub-clinical atherosclerosis risk for each subject based on the risk factors or parameters listed above. The risk point for the subject is initially set to zero at the beginning as Step 1. On Step 2, n is set to 10 to represent the number of parameters used to determine the sub-clinical atherosclerosis risk for that individual. The threshold values for the 10 parameters are stored as “Th” as Step 3. A subject supplies data for them which is stored in Step 4. In Step 5 the score points are tallied as “A[n]”. This invokes the DERIVE - SARI – POINT () function to derive the risk score point for the subject as Step 6. This function uses the parameter and threshold values to determine if any of the parameters supplied to the function contributes to increased sub-clinical atherosclerosis risk. Such individual’s risk is incremented if the parameter is determined to be a contributing risk factor. After completion of the loop, the algorithm invokes the DETERMINE - RISK – LEVEL () function as Step 7 to determine the sub-clinical atherosclerosis level for the patient. This flow chart is shown schematically in Figure 1. Subjects with risk score points between 0 and 3 are categorized as “Low Risk”, between 4 and 6 as “Moderate Risk” and 7 to 10 as “High Risk”. The FRS of the study subjects were calculated in the usual fashion utilizing age, gender, total and HDL-Cholesterol, SBP, existence of DM and being under treatment for hypertension to estimate a 10-year risk of developing CVD (11).
Table 1
Index | Decision point | Score |
---|---|---|
Age (years) | ≥39.5 | 1 point |
<39.5 | 0 point | |
AH (cm) | ≥21.75 | 1 point |
<21.75 | 0 point | |
BSI (kg/m2) | ≥37.5 | 1 point |
<37.5 | 0 point | |
SBP (mmHg) | ≥121.5 | 1 point |
<121.5 | 0 point | |
DBP (mmHg) | ≥73.5 | 1 point |
<73.5 | 0 point | |
Gender | Male | 1 point |
Female | 0 point | |
FH CVD | Yes | 1 point |
No | 0 point | |
Alcohol use | Yes | 1 point |
No | 0 point | |
PI | Yes | 1 point |
No | 0 point | |
Smoking | Yes | 1 point |
No | 0 point |
AH, abdominal height; BSI, body surface index; SBP, systolic blood pressure; DBP, diastolic blood pressure; FH CVD, family history of cardiovascular disease; PI, physical inactivity.
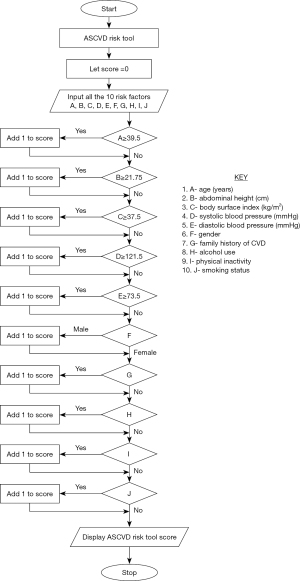
Statistical analysis
Continuous variables were subjected to descriptive statistics using SPSS (Version 21) and relayed as means (SD) as shown in Table 2; while the receiver operating characteristic (ROC) curve analysis was performed using IBM SPSS (Version 22) statistical software package. From the ROC curve analysis, we also obtained predictive cut off values used in the algorithm for the design of the App.
Table 2
Index | Mean (SD) |
---|---|
Age (years) | 36.92 (11.36) |
AH (cm) | 24.27 (4.66) |
BSI (kg/m2) | 39.82 (3.91) |
SBP (mmHg) | 123.51 (15.94) |
DBP (mmHg) | 74.80 (10.50) |
AH, abdominal height; BSI, body surface index; SBP, systolic blood pressure; DBP, diastolic blood pressure.
Cut off values used in our App for age, AH, BSI, SBP and DBP were obtained from ROC Curve analysis using IBM SPSS (Version 21). A correlation analysis was done on data on atherosclerosis risk from the 3 methods under comparison.
Results
A total of 221 apparently healthy volunteers (82 male, 139 female) were studied. Most males we contacted were not willing to participate in this study and hence the number of males reduced. Those who participated were aged between 20 and 75 years with a mean (SD) of 36.92 years (11.36). Table 1 and Figure 1 shows the atherosclerosis risk app thresholds and risk scores and the flowchart of the algorithm of Jos ASCVD risk app respectively. Table 2 shows the descriptive statistics of the indices used in the algorithms. Cut off values of the continuous variables used in the new algorithm (age, AH. BSI, SBP and DBP) were obtained from ROC curve analysis as shown in Figure 2A-2E and Table 3. For the categorical variables used, risk was ascribed to male gender, family history of CVD, alcohol use, physical inactivity and smoking.
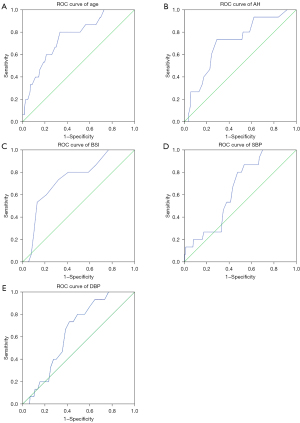
Table 3
Index | Cut-off point | AUC | Sensitivity, % | Specificity, % |
---|---|---|---|---|
Age | 39.5 years | 0.757 | 80.0 | 66.5 |
AH | 21.75 cm | 0.712 | 93.3 | 37.9 |
BSI | 37.5 kg/m2 | 0.739 | 86.7 | 34.5 |
SBP | 121.5 mmHg | 0.649 | 80.0 | 52.4 |
DBP | 73.5 mmHg | 0.645 | 80.0 | 51.0 |
AUC, area under the curve; AH, abdominal height; BSI, body surface index; SBP, systolic blood pressure; DBP, diastolic blood pressure.
The mean (SD) of the CIMT of the study population was found to be 0.053 (0.015) cm. Using the suggested CIMT cut off value of ≥0.078 cm for high risk of sub-clinical atherosclerosis (12,13), 15 out of these 221 apparently normal subjects qualified to be considered as having sub-clinical atherosclerosis. The 15 (11 males and 4 females) gave a prevalence of 6.79%. Using the designed algorithm, the total risk classifications of each of the 221 subjects who took part in this study are determined as follows:
- Low risk [0–3] =109;
- Intermediate risk [4–6] =88;
- High risk [7–10] =24.
From the algorithm, it was found that 24 participants (10.9%) were at high risk for the sub-clinical atherosclerosis against 15 (6.8%) who were at high risk based on the CIMT risk cut-off value (CIMT ≥0.078 cm) alone without considering any of the other risk factors used in the designed algorithm. However, 11 out of the 15 subjects who were found to be in high-risk group based on CIMT cut-off value are also found to be among the 24 high risk subjects identified on our new risk algorithm. This implies that 73.3% of those who are classified as high risk for sub-clinical atherosclerosis based on CIMT cut-off value ≥0.078 cm are also captured as high risk by our new algorithm. The other 4 subjects who are found to be at high risk based on CIMT were classified as intermediate risk by the new algorithm. From this new risk algorithm also, 9 subjects who were at the intermediate risk category by the CIMT method are found to be at high risk for subclinical atherosclerosis. The FRS is the oldest and most commonly used 10-year CVD risk evaluation method by many researchers [13] and its risk classification on the 221 study subjects is as follows:
- Low risk (FRS <10%) =218;
- Intermediate risk (FRS between 10% to 20%) =2;
- High risk (FRS >20%) =1.
In this method, only 3 subjects are found to be at High and Intermediate risk categories of CVD. Correlation between CIMT risk for sub-clinical atherosclerosis and our CVD risk App as well as the FRS was significant. The level of statistical significance was more for our local App (see Table 4).
Table 4
Correlations | |||
---|---|---|---|
IMT RISK | APPRISK | FRISK | |
IMT RISK | |||
Pearson correlation | 1 | 0.448* | 0.300* |
Sig (2 tailed) | 0.000 | 0.000 | |
N | 221 | 221 | 221 |
APPRISK | |||
Pearson correlation | 0.448* | 1 | 0.186* |
Sig (2 tailed) | 0.000 | 0.006 | |
N | 221 | 221 | 221 |
FRISK | |||
Pearson correlation | 0.300* | 0.186* | 1 |
Sig (2 tailed) | 0.000 | 0.006 | |
N | 221 | 221 | 221 |
*, correlation is significant at the 0.01 level (2 tailed). CVD, cardiovascular disease; IMT RISK, Risk with Intima Media Thickness score; APPRISK, Risk with New Cardiovascular Disease Prediction App; FRISK, Risk with Framingham Risk Score.
Discussion
In clinical practice, the CIMT risk is considered as the gold standard for diagnosis of subclinical atherosclerosis. It, however, requires heavy equipment and specialized manpower to determine. Again, it is not amenable for use in large scale population screening exercise or instantly in the clinical areas. The FRS, which is the most commonly used 10-year CVD risk evaluation method by many researchers requires blood-based data set. The implication again is that invasive blood sampling is required as well as equipment albeit light in the laboratories or at the point of care. The new Jos CVD risk App uses data that are generated in the clinic; not requiring any invasive procedure. At the point of care or in the field during large scale epidemiological studies the individual’s risk for ASCVD can be determined using our App installed on any smart device.
From our study as shown in Table 4, the new App correlated significantly with CIMT determined risk which is the gold standard for determining ACVD risk; and so was the FRS. The level of correlation with CIMT score was however higher for the new App than the Framingham score. The increased prevalence rate found in the new algorithm method was because it took into consideration of four traditional risk factors, age, gender and two newer obesity anthropometric indices (abdominal and general obesity) as well as the systolic and DBP. Therefore, it is believed that the sensitivity of the designed algorithm in this study is better and gives more accurate risk assessment as an entirely non-invasive screening tool of CVD. This is an improvement on the Framingham risk and also that of SCORE model (11,14) which apart from not designed for our environment, requires laboratory-derived data for computation.
In this study using the FRS, only 3 subjects (1.36%) were found to be at High and Intermediate risk categories of CVD. This does not give a true picture of subclinical atherosclerosis risk considering that it was 15 (6.79%) with the CIMT score and 24 (10.86%) with our new App. This shows in the correlations (Table 4) where both algorithm-based risk scores correlate with the CIMT, but ours (0.448, P<0.01) better than the Framingham based score (0.300, P<0.01). Other scores as reported by Marchant et al. are preferable over the Framingham score in predicting CVD risk in populations outside the USA (14). As obesity plays a significant role as one of the major risk factors of CVD, we have included two alternative obesity anthropometric indices (AH as central and BSI as general obesity index) which were found suitable in our previous publication in the Jos ASCVD App design (15).
Strengths and weakness
Our study is weak from the perspective that it is a one centre study, hence external validity is not yet determined. It was also used only on apparently normal subjects and not individuals with risk diseases. Like other algorithms, it is probabilistic tending to be only modestly accurate as newer risk factors not accommodated keep emerging.
Its strength is that it was conceived for the sub-Sahara African population whose representation in previous studies generating algorithms was scanty. It also included new anthropometric indices which appear superior to existing ones. Finally it was deliberately conceived to include data easily determinable in clinics of field without recourse to invasive blood investigations which may come at a cost hardly affordable in research constrained environments like ours.
Conclusions
Our new Jos CVD risk App has come to obviate the recourse to algorithms developed in other climes which for utilization in our environment would require re-calibration (1). It is better than the FRS which in our circumstances incorrectly categories most people into the low CVD risk group. This will under identify high disease risk individuals leading to higher rates of under-treatment and consequently high morbidity and mortality. Mis-classification of risk of a disease with such dire consequences is unacceptable. Therein lies the advantage of our new App. We are in the course of using it in patients with risk diseases to further determine its utility. We call on its wider use to further appraise this apparent benefit.
Acknowledgments
Our gratitude goes to Dr. Charles Ani who was the Head of Radiology at the time of the study for his support and cooperation.
Funding: None.
Footnote
Data Sharing Statement: Available at https://ht.amegroups.com/article/view/10.21037/ht-21-22/dss
Conflicts of Interest: All authors have completed the ICMJE uniform disclosure form (available at https://ht.amegroups.com/article/view/10.21037/ht-21-22/coif). The authors have no conflicts of interest to declare.
Ethical Statement: The authors are accountable for all aspects of the work in ensuring that questions related to the accuracy or integrity of any part of the work are appropriately investigated and resolved. The study was conducted in accordance with the Declaration of Helsinki (as revised in 2013) and was approved by the Ethics and Research Committee of Jos University Teaching Hospital, Jos Nigeria (Registration Number: NHREC/13/03/2010) in a memo JUTH/DCS/ADM/127/XIXI/6257 of 29th July 2015. Informed consent was taken from all the subjects.
Open Access Statement: This is an Open Access article distributed in accordance with the Creative Commons Attribution-NonCommercial-NoDerivs 4.0 International License (CC BY-NC-ND 4.0), which permits the non-commercial replication and distribution of the article with the strict proviso that no changes or edits are made and the original work is properly cited (including links to both the formal publication through the relevant DOI and the license). See: https://creativecommons.org/licenses/by-nc-nd/4.0/.
References
- Kullo IJ, Trejo-Gutierrez JF, Lopez-Jimenez F, et al. A perspective on the New American College of Cardiology/American Heart Association guidelines for cardiovascular risk assessment. Mayo Clin Proc 2014;89:1244-56. [Crossref] [PubMed]
- D'Agostino RB Sr, Vasan RS, Pencina MJ, et al. General cardiovascular risk profile for use in primary care: the Framingham Heart Study. Circulation 2008;117:743-53. [Crossref] [PubMed]
- Ridker PM, Cook NR. Statins: new American guidelines for prevention of cardiovascular disease. Lancet 2013;382:1762-5. [Crossref] [PubMed]
- Cook NR, Ridker PM. Calibration of the Pooled Cohort Equations for Atherosclerotic Cardiovascular Disease: An Update. Ann Intern Med 2016;165:786-94. [Crossref] [PubMed]
- Okeahialam BN, Diala UM, Uwakwe J, et al. Abdominal height measures cardiometabolic risk better than body mass index: result of a preliminary study. J Med Res 2016;2:149-51. [Crossref]
- Agbo HA, Zoakah AI, Isichei CO, et al. Cardiovascular Anthropometry: What Is Best Suited for Large-Scale Population Screening in Sub-Saharan Africa? Front Cardiovasc Med 2020;7:522123. [Crossref] [PubMed]
- Pennells L, Kaptoge S, Wood A, et al. Equalization of four cardiovascular risk algorithms after systematic recalibration: individual-participant meta-analysis of 86 prospective studies. Eur Heart J 2019;40:621-31. [Crossref] [PubMed]
- Polonsky TS, McClelland RL, Jorgensen NW, et al. Coronary artery calcium score and risk classification for coronary heart disease prediction. JAMA 2010;303:1610-6. [Crossref] [PubMed]
- Ferreira F, Duarte JA. Accuracy of body mass index, waist circumference and body surface index to characterize overweight and obesity in adolescents. Arch Exer Health Dis 2014;4:299-306.
- Sirisena AUI, Okeahialam BN, Ike EE, et al. Clinical significance of the abdominal height measured with a locally made abdominometer for risk evaluation of sub-clinical atherosclerosis. Afr J Med Physics 2019;2:15-20.
- Günaydın ZY, Karagöz A, Bektaş O, et al. Comparison of the Framingham risk and SCORE models in predicting the presence and severity of coronary artery disease considering SYNTAX score. Anatol J Cardiol 2016;16:412-8. [PubMed]
- de Groot E, Hovingh GK, Wiegman A, et al. Measurement of arterial wall thickness as a surrogate marker for atherosclerosis. Circulation 2004;109:III33-8. [Crossref] [PubMed]
- Ssinabulya I, Kayima J, Longenecker C, et al. Subclinical atherosclerosis among HIV-infected adults attending HIV/AIDS care at two large ambulatory HIV clinics in Uganda. PLoS One 2014;9:e89537. [Crossref] [PubMed]
- Marchant I, Boissel JP, Kassaï B, et al. SCORE should be preferred to Framingham to predict cardiovascular death in French population. Eur J Cardiovasc Prev Rehabil 2009;16:609-15. [Crossref] [PubMed]
- Sirisena UAI, Ike EE, Chagok NMD, et al. Alternative obesity anthropometric indices to predict subclinical atherosclerosis in adult Nigerians. Afr J Natural Sci 2020;23:13-8.
Cite this article as: Sirisena AUI, Gurumdimma NY, Oguche DE, Okeahialam BN. Development of a Jos cardiovascular disease risk app to improve screening for atherosclerotic cardiovascular diseases. Health Technol 2022;6:1.